The Future of Machine Learning Research
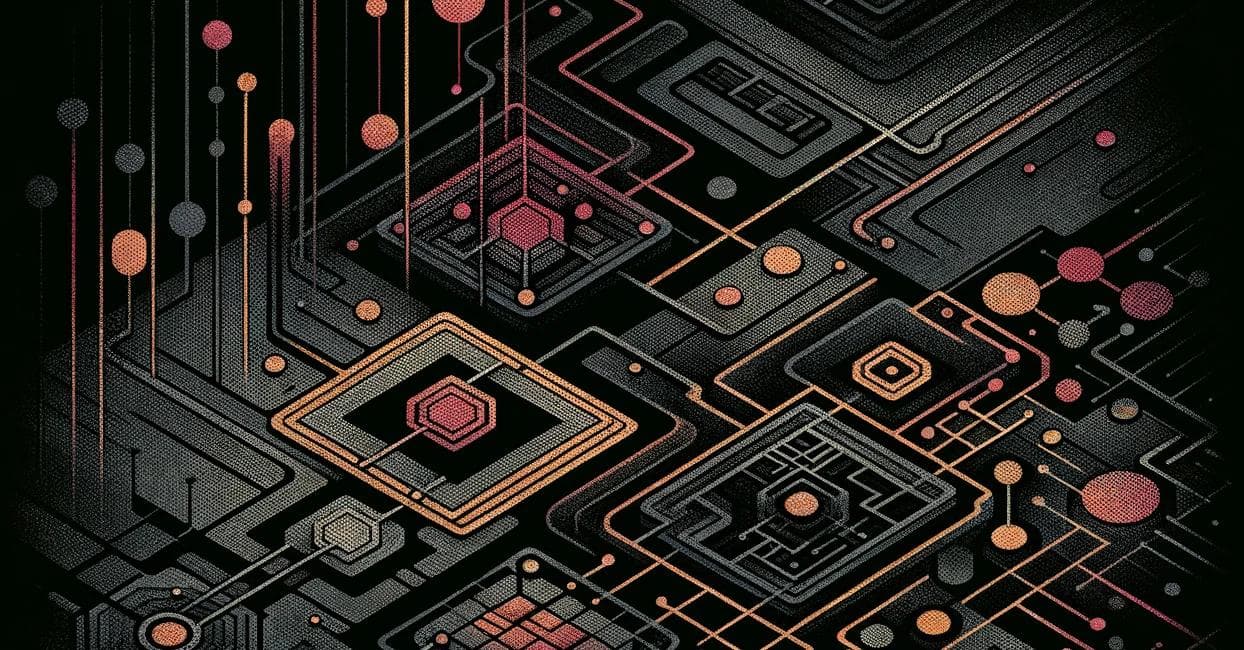
Research is a cornerstone in the quest to understand the world and tap into its economic values. At the heart of research lies the scientific method, a robust approach that hinges on empirical evidence and structured processes. This method involves hypothesizing, experimenting, observing, and drawing conclusions. It's the bedrock of acquiring knowledge that's both reliable and grounded in observable, systematic data.
At Weco AI, we are harnessing the power of Large Language Models (LLMs)-driven Agents to automate Machine Learning (ML) and Data Science research. Given the structured nature of scientific methods, automation is not only feasible but also a logical progression. LLMs have already demonstrated their capability for basic reasoning and decision making. While ML research can be challenging, much of it involves empirical trial and error, which is a good starting point for automation.
The benefits of research agents are manifold. It is fast, leveraging the inherent speed of LLMs in coding and reasoning, which outpaces human capabilities due to fundamental hardware advantages. It's cost-effective, democratizing research by lowering the traditional barriers of entry, such as hefty R&D costs that are often exclusive to large companies and academic institutions. It is scalable, allowing for the simultaneous exploration of multiple facets of a problem, using multiple agents. This scalability enables the conversion of resources, like computing power, into knowledge.
Automating Machine Learning Research
Our focus on automating machine learning (ML) research is strategic. The field of Data Science and ML is rapidly expanding, and our team possesses extensive experience in ML research. We're channeling this expertise into our AI systems, with the potential to recursively improve AI as a discipline. This improvement is not just about enhancing capabilities; it is also about fostering a deeper understanding that could lead to a safer future with Artificial General Intelligence (AGI).
Our LLM agents offer concrete benefits. They interact with users in natural language, which is more intuitive and flexible compared to conventional AutoML systems that require a learning curve to understand APIs. The design space is essentially the code space, meaning our agents can perform any task a human data scientist can, unlike conventional AutoML that operates within predefined modules or hyperparameters.
Introducing the First ML Agent: AIDE α
Our product, AIDE, currently excels at handling tabular or time series data science tasks. Users can interact with it through natural language prompts, making it intuitive and flexible tool for data scientists, machine learners, and even non-technicals. The workflow features include automatic debugging, evaluation, and iterative improvement based on previous experiments. Unlike conventional AutoML that only delivers an outcome model, our agent provides structured knowledge in the form of code and reports.
We are now onboarding alpha users. Click here to join the waiting list!
Building the Future
Our roadmap includes tackling GPU-intensive tasks, like deep learning and AI, traditionally challenging for AutoML systems due to the complexity and unstructured nature of data representations, such as images, videos, or text. The resource intensity of these tasks has been a barrier, but we're confident that LLM-driven agents can overcome these challenges.
Our ultimate goal is to achieve human expert-level performance, developing creative agents capable of novel designs and learning from cutting-edge scientific literature. We believe that AGI is not the final destination. Our research agents will continually push the boundaries of human knowledge, driving technological innovation at an unprecedented pace. Join us on this journey to build the future of research!